Breaking Down Willingness-to-Pay in Airline RM
by Justin Jander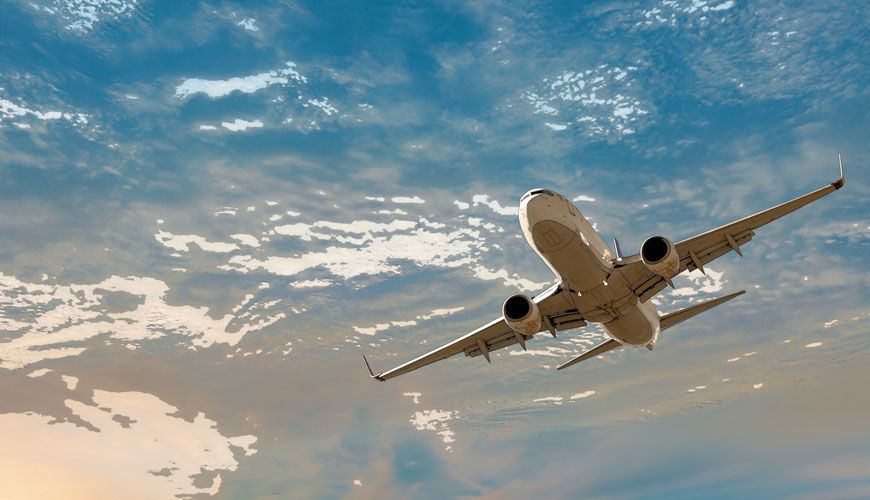
Why Willingness-to-Pay is Changing Airline RM
By the classical definition, the goal of airline revenue management is to sell the right product to the right passenger at the right price. Over the 35+ years since the formalization of revenue management, there have been many different approaches to solving this problem. The key focus areas to successfully solving this problem have always been forecasting the passenger demand, valuing the passenger demand, and then performing an optimization to determine the right controls for an airline’s remaining inventory of seats. Those fundamentals have persisted with different approaches to all three areas since revenue management has been practiced.
Willingness-to-Pay (WTP) forecasting and optimization science is revolutionizing airline RM by helping airlines forecast and price based on customers’ price sensitivity, not class availability.
How to Prevent Buy-down in Airline RM
Willingness-to-Pay forecasting is an industry-first technology that helps airlines scientifically combat buy-down and drive incremental revenue. Buy-down is the concept where passengers have a certain willingness-to-pay for a product but will buy down to the lowest available class. This effect dilutes the airline’s revenue unless protections are in place to prevent the buy-down. In an environment with few competitors or fenced fare structures, the buy-down is automatically controlled through the implicit segmentation in the filed fares. However, as the airline industry has moved away from the traditional fencing, more input is required to control the availability to prevent buy-down. Taking this approach allows for a more scientific approach to something that was previously handled through manual intervention.
In 2007, PROS pioneered the science to combat buy-down by introducing Hybrid Forecasting and Optimization. Hybrid opened the door for airlines to begin addressing this problem, and an earlier version of this science quickly proved successful, helping one airline increase revenue in three key markets by 4.7%, with the six routes generating an additional US$1.5 million in fare revenue. Read the full case study here: Willingness-to-Pay Solution Recaptures Revenue with Leading Science.
PROS has continued the evolution of this approach by implementing the PROS Willingness-to-Pay (WTP) Forecasting and Optimization methodology. This guide outlines the key steps to the WTP methodology:
- Forecasting the price sensitivity of the demand;
- Adjusting the value of the passenger to account for the dilution through an optimization procedure.
Overview of Willingness-to-Pay Based Revenue Management Practice
In the early days of revenue management practices, the class codes (RBDs, classes, Fare Class, etc.) were the primary indicator of segmentation of a passenger’s willingness-to-pay, or price sensitivity. The class represented the “product” that the passenger wanted to purchase, and the price associated with the product. The airline filed fares for each of the classes based on the passenger’s price sensitivity and for the conditions associated with the fare. Those conditions made up the particular product that the passenger wanted to buy. These were tangible conditions like flexibility to cancel or refundability of the ticket. They were also conditions like the number of days before departure that the ticket was purchased. These conditions, and others, were designed to create segmentation amongst the passengers. In some cases, these practices are still being used. The passenger’s choice of a particular product could be a passive or active decision. In some cases, the passenger is actively choosing the conditions of the fare, while in other cases, the number of days before departure a passenger was buying made them unaware, they were choosing the product and thus a passive decision.
The traditional revenue management system forecasts the passenger demand at each of these classes and then optimizes under the assumption that the demand is purely interested in buying that class code due to the restrictions and conditions of the fare. The resulting optimization produces controls, typically bid prices, that set the lowest available class that should be sold based on the constraints to capacity imposed by the expected demand and value of that demand. This is done by comparing the fare for the class to the bid price, which serves as a hurdle rate.
However, with airlines moving to digital distribution of prices and low-cost carriers (LCCs) entering more markets, there has been a more targeted effort to remove the fare fences that were traditionally visible to the airlines. Removing this segmentation results in class codes having the same product association, but at different price points. Thus, the buy-down effect is observed as the passenger will only choose the lowest available class. As this problem has become more prevalent, airlines have begun using different phrases like price elasticity, price sensitivity, buy-up, buy-down, trade-up, yield-up, spiral down, class dependence problem, etc. All of these terms represent the situation that is being faced and highlight the need to address it. To combat this situation, airlines have employed different approaches. In most cases, airlines choose to use a rules-based approach in combination with the traditional forecasting and optimization. Using this combined approach, the bid price sets the lowest available class based purely on the capacity constraints, and then the analyst creates an action or rule that closes the lower classes as the departure date nears, forcing the demand to buy-up to those classes, preventing dilution.
In some cases, the analyst may also employ a load factor rule that will close the lower classes as the load factor increases. Airlines may also combine these approaches as well.
This approach has proven valuable at airlines, particularly those that take a method-driven approach to creating the rules. However, the application of these rules can often be too broadly or improperly defined, causing close-off of too much or too little. Further, this approach can also impact the network effect by overriding the result of the network optimization. And, because of the significant manual effort this approach requires, airlines leave themselves open to errors in inputs or missed opportunities.
Another approach airlines may employ is a methodology that increases the demand in the higher classes, closer to departure. This gives the traditional optimization the impression that higher-paying demand exists closer to departure, potentially setting controls at a higher availability level. The assumption with this approach is that there is enough demand for those classes willing to pay that fare. However, this approach still relies only on the bid price to force the buy-up, which is not the intended use for it. If a flight does not have enough demand to fill the plane, the bid price is likely to be quite low, indicating that any fare is sellable.
In this case, there is potentially still revenue opportunity by forcing demand to buy into the higher classes, which won’t be accomplished using just the bid price.
The ideal revenue management methodology for handling this business environment consists of forecasting and optimization under the consideration of this buy-down scenario occurring. The first step is to forecast the price sensitivity based on the passenger’s willingness-to-pay. The second step is to use this price- sensitive forecast to apply a marginal revenue optimization to account for that willingness-to-pay. Once that optimization is complete, the network optimization can follow, along with the dynamic program to calculate network optimal bid prices. After the revenue management processes are complete, the availability calculator receives this transformed fare as well as the bid prices, which are used to calculate availability. The PROS RM Advantage solution, Willingness-to-Pay (WTP) Forecasting and Optimization, along with PROS Real- Time Dynamic Pricing (PROS RTDP), have these steps fully integrated in the solution (figure 1).
The remainder of this guide is a deep dive into the PROS recommended approach to forecasting and optimization with WTP methodology.
Figure 1 Outline of the key steps in the process along with PROS approach to solving those steps.
Understanding Willingness-to-Pay Forecasting
The PROS RM Advantage forecasting methodology is based on the Bayesian Hierarchical model. The implementation of these concepts was done by PROS specifically for the airline demand forecasting environment. It has been further extended to other industries through other PROS products. It continues to outperform other methods for forecasting. The key design considerations of the PROS Bayesian model are the following:
“Drift” Setting
- Controls the speed at which the system learns from new observations
- Helps detect between noise and new signal
Data Collection Points (DCP)
- Ranges of days prior where the demand arrives uniformly
- Each DCP is forecasted separately to determine the expected arrivals within that period
DCP Correlation
- The relationship between the DCPs
- This setting is used to determine the amount of sharing across the DCP periods
Seasonality
- Automatic capturing of seasonality as a covariate in the model
- The seasonal shape of the historical observations is captured as part of the model, not requiring any multiplicative factors to be applied
Holidays and Special Events (HSE)
- Periods during unusual demand patterns
- These periods are excluded from the base forecast, so the observations do not impact the remaining periods
- A multiplicative factor is calculated based on the impact the HSE period will have on the base forecast.
With the framework established in the Bayesian model, it serves as the baseline methodology for forecasting passenger demand within the PROS RM Advantage product. The next step in the process is using this framework to forecast the price sensitivity of the demand. In the PROS WTP model, the system assumes that all demand within a set of classes (called a class block) is price sensitive. This means that within a given set of classes, all the demand will buy down to the lowest available class. The definition of the set of these classes, called a class block, is based on criteria set by the airline, where the only difference between the classes is the price. The block represents the product, while the classes represent the price points for the product. Once these are established, the system will generate the price sensitivity at the class level. This configuration is completed during the implementation of the WTP model.
In the PROS RM Advantage product, the price sensitivity of the demand is modeled as an exponential price/ demand curve. In this formulation, demand is a function of price with two parameters to control the shape and height of the exponential:
The lambda parameter estimates the volume component of the function and the alpha parameter represents the shape of the curve. Alpha also represents the amount of price sensitivity in the demand (figure 2). The p0 is a minimum price in the market.
Given this formulation, the next step is for the PROS Bayesian forecaster to generate a forecast of the alpha and lambda parameters. This is done by first generating historical observations of the alpha and lambda values. In a traditional bookings forecast, the observations are the bookings and a constraint probability, which is used to calculate an unconstrained bookings observation. These unconstrained bookings are used as an input into the model. In the WTP model, the alpha and lambda parameters are not directly observed, but instead are generated based on the historically observed bookings and the price paid for those bookings.
Figure 2 Representation of the parameters of the exponential demand curve.
In PROS RM Advantage, the system associates every booking observation with the price paid. In order to accomplish this in RM Advantage, the system will use the daily fare that was in place at the time the booking occurred, as well as the daily fare offered during periods where no bookings occurred. Using the bookings with the associated fare allows the system to focus on the expected demand at different price points, rather than being associated with the fare class itself. This is the first step in the process where the RM system is breaking away from the barriers of the class codes. Once the PROS Bayesian model has generated the alpha and lambda forecasts, the system estimates the cumulative price-sensitive demand at each of the current class codes that make up the class block by discretizing the curve at the current fare value (figure 3).
Figure 3 Price Demand curve after discretization to the class level.
This approach provides several key characteristics that make it a robust model for estimation of the price sensitivity. Those are:
- Using the historical fares rather than associating the price sensitivity to the class letter directly
- No reliance on the actual availability data to estimate the price sensitivity
- Availability data can be unreliable and may only be estimated once per day
- More flexible to fare class realignments
The product is represented by the model, which is independent of any class codes. Should the class codes in the block be changed, the model state for the product (block) remains unchanged and can be associated with the new classes added to the block. - More flexible for fare adjustments
When fares are adjusted, the continuous curve allows for interpolation between the previous fares, which provides a more robust estimation of the price sensitive demand.
The output of this process is an estimation of the amount of price sensitive demand at the following level:
- Departure Date
- Online O&D
- Trip O&D
- POS
- Itinerary
- Fare Class
- DCP
This forecast is then sent to the optimization process for fare transformation.
Applying Willingness-to-Pay Optimization
In PROS RM Advantage, there are three key steps to the optimization process:
- Demand/Fare Transformation (marginal revenue transformation)
Generates WTP-adjusted fares - Network Optimization (Linear Program)
Generates the displacement costs to account for the network effects - Leg Optimization (Dynamic Program)
Generates the bid prices based on the fares and displacement costs from the previous two steps
PROS RM Advantage uses the properties of the network optimization and dynamic program where the transformed fare and demand can be used, without any changes to the traditional formulation. This means that the primary focus on the WTP Optimization is the calculation of the transformed fares and demand.
The transformation process is a marginal revenue calculation called the Concave Envelope Data Transformation (CEDT). The algorithm assesses those fare classes that are on the efficient frontier. An efficient class, one on the efficient frontier, is one that should be open under at least one available capacity scenario to maximize revenue. A class lying below the efficient frontier, called inefficient, is a class that should never be open for sale under any available capacity scenario and thus the class is closed or inherits the availability from the class below it. The intention of this process is to identify classes where the revenue is diluted so much that it results in a negative margin, thus in a revenue maximization process, it is optimal to close the class. Once this step of closing classes has occurred, the system next performs a demand and fare transformation. The purpose of this step is to provide an estimation of the actual amount of demand expected at each class and the relative value of that class, given the incremental revenue achieved in that class. The transformed demand is calculated as the incremental demand if one additional class is open for sale. The transformed fare represents the incremental revenue per passenger if one additional class is open for sale. Once the transformed fare and demand are calculated, they are sent to the Linear Program for network optimization and the dynamic program for leg optimization. The resulting outputs from the optimization are the flight/leg/compartment bid prices and the ODIF/ POS level transformed fares. Figures 4-6 show an example of this calculation of transformed fares and demand.
Figure 4 The demand (as calculated from the forecast), daily fare, and calculated cumulative revenue. These represent the input into CEDT.
Figure 5 Visual representation of the efficient frontier. Class V lies below the efficient frontier and thus is inefficient.
Figure 6 Transformed demand and fares calculated. Since V class is inefficient, the transformed fare is $0.
The final step in the process is to perform an aggregation of the transformed fares to bring them closer to the level of the daily fares. This step is performed in order to reduce the number of transformed fares created. PROS RM Advantage has a variety of aggregation options available, which can be configured in the system.
Since the transformed fare is the representation of the incremental revenue for a given class, this is now used when comparing a fare to the bid price for the purposes of determining availability. PROS RM Advantage outputs the necessary data for consumption by the availability calculator. PROS RTDP Advantage is seamlessly integrated with RM Advantage, allowing the system to send real-time updates of new bid prices to RTDP. Once this data is in RTDP, the availability is calculated by comparing the transformed fare to the bid price. If RTDP does not find the transformed fare, the system falls back to the daily fare provided by RM Advantage.
WTP Benefits: Scientifically Proven Revenue Uplift
As the airline industry continues to evolve to keep up with market shifts and competitive environments, airline revenue management systems must evolve and modernize with it. One of the biggest evolutions related to revenue management is the way the price and product are presented in the market and how the price sensitivity of the passenger is considered. Airlines have approached this with unscientific, rules-based methods, which at best prevent significant revenue dilution and at worst cause more revenue dilution.
The solution to this is to perform scientific forecasting and optimization steps to best capture the price-sensitive demand and adjust availability accordingly. With PROS WTP methodology, PROS has built upon previous experiences from traditional and hybrid forecasting and optimization to build the next stage in modernizing airline revenue management. The WTP model uses the observed bookings and the fare of those bookings to estimate a continuous price/demand curve. These forecasts are then discretized to the class level and sent to optimization where optimal controls can be generated. This data is seamlessly integrated with PROS RTDP to distribute accurate and timely availability.
The benefits of PROS WTP Forecasting and Optimization include both revenue improvement as well as more flexibility in the model for the analyst in the changing business environment. The revenue improvements have been studied using both simulation methods as well as with real data.
This solution has proven benefits in both simulation and with real airline data. The results consistently show an improvement of between 1% and 3% over traditional revenue management controls where the fare classes are assumed independent.
The improvement is on top of the benefits already achieved by moving to O&D-level forecasting to take into account the network effect.
The revenue benefits will be dependent on the market characteristics, including the competitive environment, fare fences/conditions, and the price sensitivity of passengers. Regardless, Willingness-to-Pay methodology unveils great revenue potential for airlines to exploit on the path to class-free dynamic pricing and modern airline retailing.